TL; DR
Qualitative and quantitative data are both crucial.
Debates over which is better hold back progress but are sadly all too common. Some "qual" and "quant" people even pay lip service to the other "side" but still do nothing at all to embrace the other kind of data (and people) actively.
The solution? Truly value and then use both, typically sequentially, first qual to understand reasons and then quant to learn nuances at scale.
Why qual vs. quant causes arguments
At the heart of the debate is a fundamental disagreement over what counts as “real” or “good” data.
Advocates for qualitative data emphasize the depth of insight—the ability to capture the “why” behind user behavior and motivations. On the other side, quantitative data champions value concrete metrics that indicate “where,” “when,” and “to what degree” things occur.
Each camp sees the other as focusing on the less-important part (at best) or outright bozos, who don't know what matters (at worst). When that happens, qualitative enthusiasts see quantitative methods as too superficial. Meanwhile, quantitative supporters berate qualitative approaches as subjective and anecdotal.
This rigid divide stalls progress and hurts relationships. You can't innovate credibly if you fall prey to it. And you look a fool to everyone else to boot.
Why the argument Is silly
You can't win with just one data type. Treating qualitative and quantitative data as mutually exclusive ignores the strengths they both offer, which–it turns out–you both need. Successful teams understand that blending both approaches gives you more complete understanding of any issue.
We could describe what qual and quant data are at length. But, just keeping things very simple, qualitative data in innovation often unlocks the stories, patterns, and user motivations that spark new ideas and hypotheses. Quantitative data then lets you test these insights, measuring their nuances, and estimating their impact at scale. One without the other leads to either uninformed ideas or data-driven decisions with no context. Conversely, having both unlocks all the insights available to you.
For example: One of my favorite experiences at a client was when I walked into the office and saw a very senior designer having a thoughtful conversation with a data scientist. Yes, the designer's expertise was on the qual side of things. But he understood enough about quant data to have a meaningful work discussion about it. And, more important, he valued the data scientist and their work (and vice versa) enough to make this conversation a priority.
A Better Way
The key to credible innovation then is thoughtful, respectful integration.
Normally, start with qualitative exploration to discover user pain points and understand motivations. Use these insights to inform hypotheses. Then, transition to quantitative validation to gauge how widespread and significant these findings are. This combined approach gives you a foundation that teams and stakeholders can actually trust.
How to Do This – A Flowchart
The following flowchart gives you an example of this transition from qualitative exploration to quantitative validation. It does so using the topic of user (Desirability) understanding. But it applies similarly to other fields, across DVFA.
Said simply, follow this scale, and you'll pretty much know for certain how close you are to having a Lovable solution:
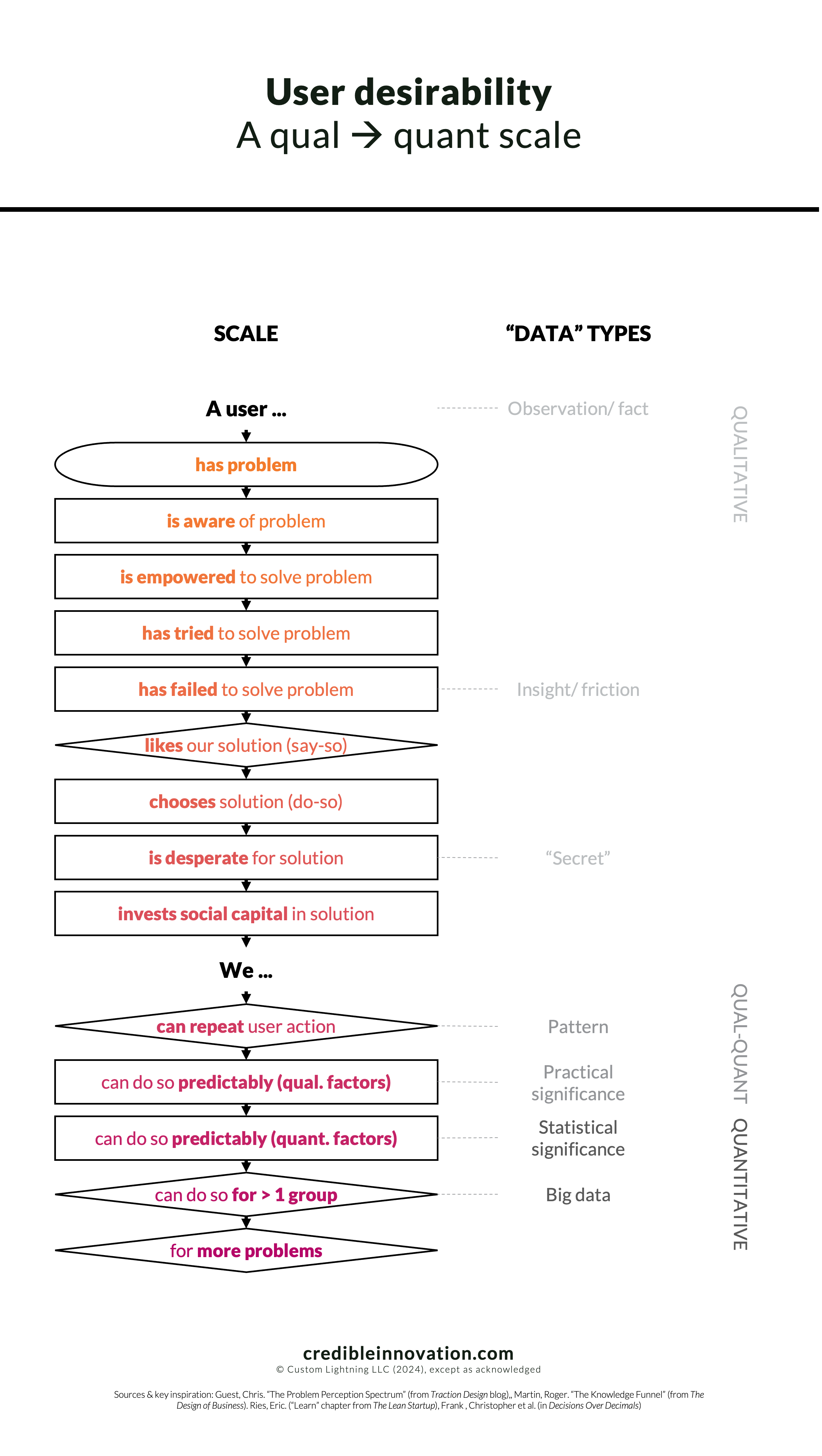
Of course, this builds on the work of others, even though this particular version is the one I have built for my in-project use.
In particular, it builds on Chris Guest's “Problem Perception Spectrum” (from Traction Design blog), Roger Martin's “Knowledge Funnel” (from The Design of Business), Eric Ries's (“Learn” chapter from The Lean Startup), and Christopher Frank et al's integration of intuition and information (in Decisions Over Decimals). You can (and I do) even map Safi Bahcall's 3 Deaths (from Loonshots) to the same scale. Thanks to them all!
But on to this version. I created it because I needed something that led continuously from the earliest qual insights to the most rigorous quant data, monkey bar-style.
Let's look at each stage of the flowchart in turn.
Qualitative Phase: Understanding the User Problem to a Solution
- User has a problem - Here, our qualitative data is a simple observation
- User is aware of the problem
- User feels empowered to solve the problem
- User has tried to solve the problem
- User has failed to solve the problem - This is often the stage where we start to talk about "insight"-type qual data. (Note: This stage is also really important. There only is a point in keeping going if users have both tried and failed at finding a solution.)
- User likes our solution (expressed through feedback – "say-so")
- User chooses our solution (through action – "do so")
- User is desperate for the solution - This is often where we know something important that opens an opportunity, a "secret" using the term I believe I heard attributed to Peter Thiel
- User invests their social capital in the solution (e.g., tell their friends about it)
Qual-Quant Phase: Expanding with Mixed Data
These phases often involve qualitative data that begins to include initial quantitative measures, but often not (yet) at the scale needed for broad generalization.
I have shifted the tone from "the user" to "us" at this point because we really start to think of all users, no longer an individual one here. Our insights must still be grounded in reality and good data collection and analysis of course. But it's still no longer as much about each individual users.
- We can repeat user actions
- We can do so predictably (based on qualitative factors) - Here we have "practical significance" that isn't statistical yet but clearly trends in a direction that is on the way to it. There isn't really an official "end" to what makes for "qual-quant" data. But once patterns start to become nuanced and concrete turns out to be a good practical cutoff in many cases.
Quantitative Phase: Generalizing Broadly
This stage ensures comprehensive insight into user desirability toward product-market fit.
- We can do so predictably (based on quantitative factors) - This is where we often reach statistical significance.
- We can apply findings to more than one user group - Many times, this is where big data starts to be in use. We are now in ultra-heavy quant territory
- We can apply findings to additional problems
Go Do
Use both data types
Teams that strategically employ qualitative and quantitative data are basically just better innovators. They know why what is true when and how.
So next time a “qual vs. quant” debate surfaces, remember that making peace with both camps is the key. Start integrating small elements from both, iterate, and refine your approach.
Value "the other kind" of people
And don't just consider both types of data. Consider both types of people, meaning people who understand (or even value) one better than the other.
Create your own scales for other topics
Our flowchart about Desirability above is not unique, just one in a quasi-infinite set of options for all kinds of topics. Consider what topics matter to you and agree with stakeholders on scales that make sense in your world.
Overall, remember: Credible innovation isn’t about choosing one or the other—it’s about using both to build better products and services.
Further reading:
Bahcall, S. (2019). Loonshots. St. Martin’s Press. https://books.google.com/books/about/Loonshots.html?hl=&id=b55xDwAAQBAJ
Frank, C. J., Magnone, P. F., & Netzer, O. (2022). Decisions Over Decimals: Striking the Balance between Intuition and Information. John Wiley & Sons. http://books.google.com/books?id=eMiAEAAAQBAJ&hl=&source=gbs_api
Martin, R. L. (2009). The Design of Business. Harvard Business Press. https://books.google.com/books/about/The_Design_of_Business.html?hl=&id=CvpAgm8dQQkC
Ries, E. (2011). The Lean Startup. Crown Books. http://books.google.com/books?id=r9x-OXdzpPcC&hl=&source=gbs_api